Empowering researchers to achieve more
How do convolutional neural networks (CNNs) in AI-boosted mammograms differentiate between benign and malignant features?
CNN AI mammograms differentiate between benign and malignant features through various approaches directly from mammographic images eliminating the need for handcrafted feature engineering
2/15/2025
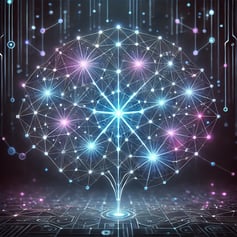
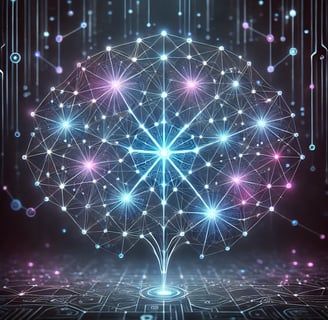
How do convolutional neural networks (CNNs) in AI-boosted mammograms differentiate between benign and malignant features?
Convolutional Neural Networks (CNNs) have shown significant potential in differentiating between benign and malignant features in mammograms through various approaches:
CNNs can automatically learn and extract relevant features directly from mammographic images, eliminating the need for handcrafted feature engineering [1,2]. These deep learning models can be trained on large datasets of mammograms to recognize patterns and characteristics associated with benign and malignant lesions. Transfer learning techniques have been particularly effective, allowing CNNs pre-trained on large-scale natural image datasets to be fine-tuned for mammographic analysis, achieving high accuracy and area under the curve (AUC) scores [3,2].
Interestingly, some studies have found that combining CNN-extracted features with traditional computer-extracted tumor features can significantly improve classification performance. For instance, reports that ensemble classifiers using both CNN and analytically extracted features outperformed classifiers based on either type alone, achieving an AUC of 0.86 compared to 0.81 for individual classifiers [4].
In conclusion, CNNs have demonstrated superior performance in differentiating between benign and malignant features in mammograms by leveraging their ability to learn complex patterns directly from image data. The use of transfer learning, feature fusion, and ensemble methods has further enhanced their effectiveness, making CNNs a promising tool for improving computer-aided diagnosis (CADx) systems in breast cancer detection and classification [5].
Références
1. Huynh BQ, Giger ML, Li H. Digital mammographic tumor classification using transfer learning from deep convolutional neural networks. Journal of Medical Imaging. Spie; 2016;3:034501.
2. Liu, Y., Pu, H., & Sun, D. W. (2021). Efficient extraction of deep image features using convolutional neural network (CNN) for applications in detecting and analysing complex food matrices. Trends in Food Science & Technology, 113, 193-204.
3. Tsochatzidis, L., Koutla, P., Costaridou, L., & Pratikakis, I. (2021). Integrating segmentation information into CNN for breast cancer diagnosis of mammographic masses. Computer Methods and Programs in Biomedicine, 200, 105913.
4. Qureshi, S. A., Hussain, L., Sadiq, T., Shah, S. T. H., Mir, A. A., Nadim, M. A., ... & Shah, S. A. H. (2024). Breast Cancer Detection using Mammography: Image Processing to Deep Learning. IEEE Access.
5. Li H, Nailon WH, Chen D, Davies ME, Laurenson DI. Dual Convolutional Neural Networks for Breast Mass Segmentation and Diagnosis in Mammography. IEEE Transactions on Medical Imaging. Ieee; 2022;41:3–13.