Empowering researchers to achieve more
Challenges in implementing AI-boosted mammography on a larger scale
Challenges in implementing AI-boosted mammography on a larger scale. AI-boosted mammography faces several key challenges for large-scale implementation in healthcare systems
3/1/2025
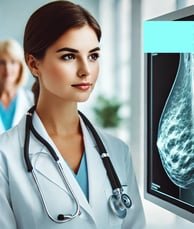
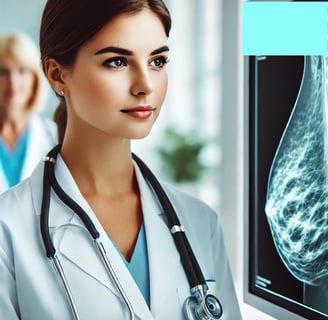
What are the key challenges in implementing AI-boosted mammography on a larger scale in healthcare systems?
AI-boosted mammography faces several key challenges for large-scale implementation in healthcare systems:
Radiologists and radiographers identify breast imaging as the subspecialty likely to be most impacted by AI, with mammography being one of the primary modalities for AI application [1]. However, integrating AI into clinical practice encounters significant hurdles. A major barrier is the lack of mentorship, guidance, and support from AI experts, hindering the learning process for healthcare professionals [1]. This knowledge gap can impede effective implementation and utilization of AI tools in mammography.
The adoption of AI in healthcare, including mammography, is further complicated by issues such as biased and heterogeneous data, data management burdens, insufficient clinical validation, and workflow challenges [2]. Additionally, outdated regulatory and legal frameworks pose obstacles to widespread implementation [2]. These factors collectively contribute to the slow adoption of AI tools in clinical settings, despite their demonstrated technical performance in image analysis and predictive analytics.
In conclusion, successfully implementing AI-boosted mammography on a larger scale requires addressing multiple challenges. These include providing adequate training and support for healthcare professionals, standardizing data and validation methods, updating regulatory frameworks, and fostering multidisciplinary collaboration [2]. Overcoming these barriers is crucial for harnessing AI's potential to enhance breast cancer detection and improve patient outcomes in healthcare systems.
References
1. Al Mohammad B, Gharaibeh M, Reed W, Aldaradkeh A. Assessing radiologists’ and radiographers’ perceptions on artificial intelligence integration: opportunities and challenges. The British journal of radiology. British Institute of Radiology; 2024;97:763–9.
2. Chua IS, Korach ZT, Kehl KL, Hassett M, Jackson GP, Arriaga YE, et al. Artificial intelligence in oncology: Path to implementation. Cancer Medicine. Wiley-Blackwell; 2021;10:4138–49.